The Growth Leader’s Playbook for Scalable Experimentation
The frameworks, docs, and lessons for running high-impact growth experiments
Dear GTM Strategist!
Every week, I talk to 20+ entrepreneurs, VCs, consultants and business ecosystem leaders. There is one question that I ask at the end of every call:
“What is the hardest thing in your business?”
And lately, the most frequent answer to this question has been growth.
Budgets are getting tighter, uncertainty is suffocating investments, and AI has accelerated the law of diminishing returns, eroding ROI on channels and tactics that used to work very well.
Getting something to work is harder than ever.
Scaling something exponentially more so.
Not to be too pessimistic, whenever business seems stuck, I like to return to the essentials: are we purposefully solving the problem and have a system that enables us to learn, or are we scattering experiments all over the place in hopes of hitting the jackpot?
I invited Andrew Capland, PLG advisor who helped over 50 teams , from early-stage startups to $100M+ SaaS companies, including Copy.ai, Sprout Social, Pendo, Lusha, and Wistia to get unstuck on their growth journey.
In this post, he’ll break down the exact frameworks, docs, and rituals that have worked again and again across different companies, stages, and team setups.
This guide is for growth leaders, marketers, and product teams tired of scattered tests and shallow results. You’ll learn how to:
Spot and prioritize high-leverage ideas
Test faster and smarter
Build a learning loop that compounds
Make experimentation second nature
But first - why do most teams get stuck in the “just run more tests” phase?
This post is sponsored by Tidio. Tidio’s customer support AI agent, Lyro, guarantees a 50+% resolution rate with hassle-free setup and no strings attached.
Installed by over 300,000 businesses, Tidio is a complete customer service platform that consolidates your support requests from live chat, email, and social media into one dashboard.
See Lyro AI Agent in action in a live sandbox:
Why experimentation is so important
Experimentation is the process of learning and iteration.
The inputs to eventually figuring out what works, and scaling your business.
In the early days of a company, success is often measured by shipping. This is typically called the 0-1 stage of growth. You need to build the first version of many of the core components of the business:
Product positioning
Acquisition channels
New user onboarding experience
Pricing and packaging
Checkout and upgrade flows
Etc
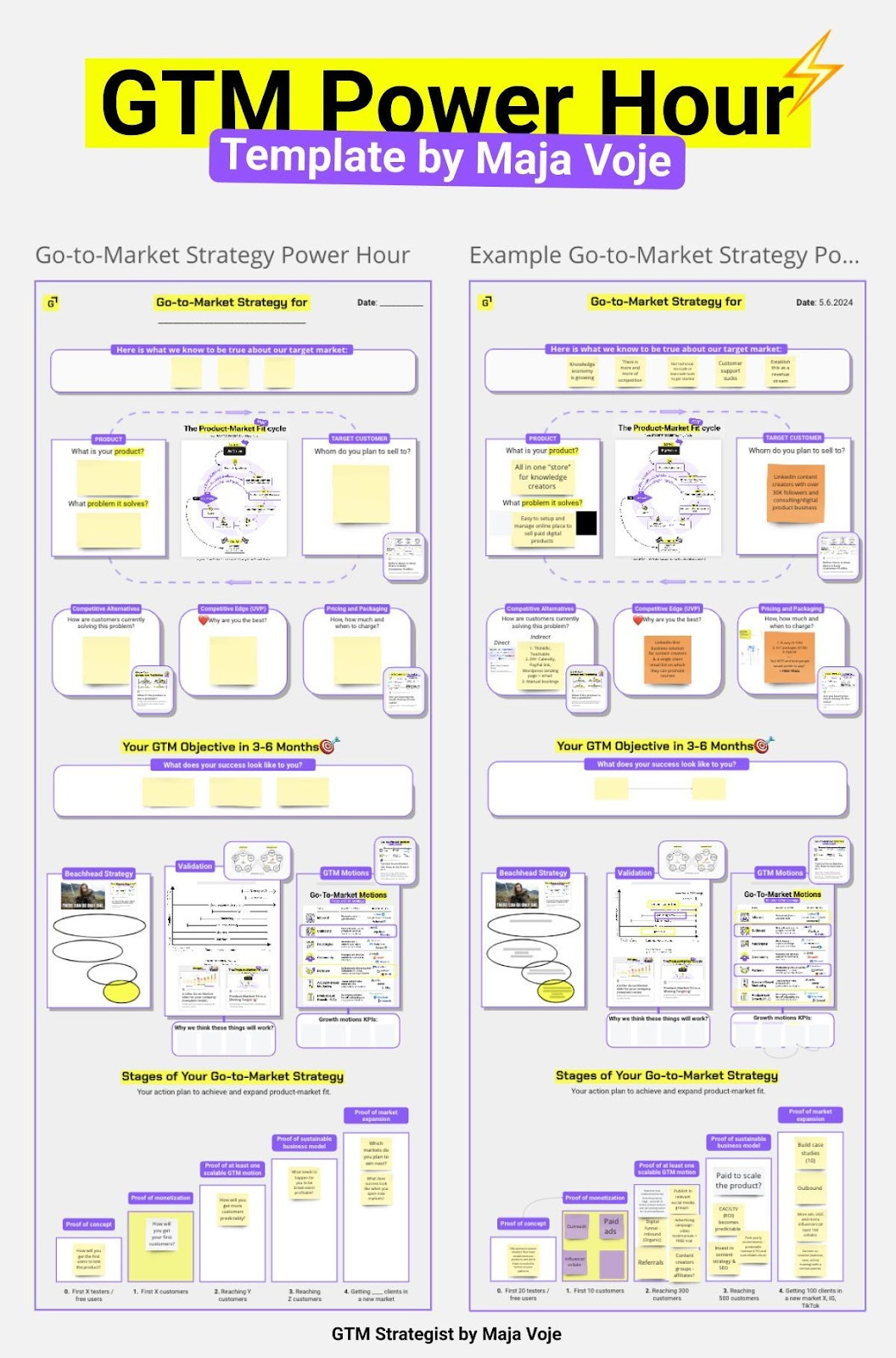
But once those first versions are live, that’s when the real work begins. Because what got you here, won’t get you to the next stage.
To grow faster, you need to figure out which parts of your experience are working… and which ones are holding you back. Then iterate and improve those experiences to help you break through plateaus.
That’s where experimentation comes in.
It’s not about testing for testing’s sake. It’s about learning what works, and what doesn’t. And to do it correctly, you need a system. A way to identify where you should test, how to brainstorm impactful ideas, prioritize them based on their potential, and then learn what works/doesn’t. A way to turn ideas into results.
And the faster you can learn what works/doesn’t - the faster you can grow.
The 4 main components of a high-impact experimentation program
You can’t actually learn what works (at scale) without a clear system behind it.
You can’t just throw ideas at the wall and hope something sticks. You need a repeatable process. One that helps you spot opportunities, generate strong ideas, prioritize what matters, and ship consistently.
Let’s walk through each part of that system.
1. Find the bottlenecks in your growth model
A good experimentation program starts by knowing where to focus.
Start by zooming out.
Look at your entire growth model from acquisition to activation, retention, and monetization. Ask yourself:
Where are the major drop-offs?
Which areas aren’t converting well?
Which areas are underperforming?
Where are you lacking volume - or quality?
… and if you could fix those things, which will have the biggest impact on your business?
This is the time to merge quantitative and qualitative insights.
Dig into your analytics to spot trends and drop-off points—then go deeper using user interviews, session replays, support tickets, and in-product surveys. Quant tells you where users struggle. Qual helps you understand why.
You need both.
It’s a mistake to jump into testing without first doing this diagnosis work.
When you skip it, you end up solving the wrong problems.
2. Brainstorm 10% tweaks and 10x swings
Once you’ve identified the bottlenecks, it’s time to start brainstorming ideas.
But not all ideas are created equal. Some are safe, obvious, incremental. Others are bold, creative, and high-upside.
This is where I use 10% vs 10x thinking.
Ask yourself (and your team): “What’s a 10% improvement we could make?” Then: “What’s a 10x change that could reinvent the experience?” The tension between those two lenses surfaces stronger ideas.
For example, let’s say you’re a B2B SaaS company looking to increase your purchase rate.
Ideas that might result in a 10% improvement in purchase rate might look like:
Updating the H1 on the pricing page
Adding FAQ copy at various points in the checkout flow
Improving the nurturing for users who have visited the pricing page, but not converted yet.
Ideas that might result in a 10x improvement might look like:
Changing the price of the product from $199 to $99
Making the free plan less generous
Shifting from a free plan to a free trial (or reverse free trial)
Don’t just settle for color changes and button copy. Push your team to think bigger.
That’s how you stumble into the experiment that changes everything.
3. Prioritize ruthlessly with ICE
Once you have a list of ideas, the next step is deciding what to test first. This is where teams can spin out, because everything feels important.
The ICE framework gives you a way to compare apples to apples.
Score each experiment by Impact, Confidence, and Ease (on a scale of 1–5), then calculate the average. This forces you to weigh your bets based on potential, not emotion, excitement, or seniority.
The best teams are disciplined here. They don’t chase every idea. They find the right ones.
4. Create a roadmap with quick hitters and big swings
A good roadmap is balanced.
You need some quick wins. Experiments that are fast to build, easy to ship, and likely to move the needle. But you also need big swings. Bets that take longer but could unlock transformational growth.
If your roadmap is all quick hits, you’re probably playing too small.
If it’s all big bets, you’ll burn out before you see any wins - or it’ll just take forever.
Mix the two. And always build from a shared doc. I recommend a centralized backlog with your backlog of ideas, current tests in progress, and key learnings, so the whole team can stay aligned and see the compounding impact of your work.
Let’s explore docs next.
The docs that power your experimentation program
We’re not documenting for the sake of it.
That’s how you drive visibility, alignment, and (most importantly) speed. There are three core docs every growth team needs.
They’re the operating system behind the scenes.
1. Experiment backlog
This is your single source of truth for all potential tests that your team(s) have brainstormed.
You can do this in a project management tool, but I personally like to use a spreadsheet tool. Each row should include:
What area of the growth model are we testing
A description of the test
Whose idea was it (in case we need to ask clarifying questions later)
A clear hypothesis on why we’re excited about the idea
The target success metric or part of the funnel
An ICE score (Impact, Confidence, Ease)
Status (not started, in progress, completed, etc.)
Link to the full experiment doc
When clients bring me in to help build out their growth function, this is one of the first docs I ask to see.
Not because I want to judge their ideas.
But because it tells me everything I need to know about how they work.
2. Experiment docs
This is where you define what you're testing, why, and keep tabs on the details.
Here’s what I recommend including:
Hypothesis: we believe that….
What we’ll learn from this test:
If our hypothesis is right: What do we learn? Where else can we apply this learning?
If our hypothesis is wrong: What do we learn? What other hypothesis do we have?
Relevant background information
Main success metric:
Secondary metrics:
An overview of the test:
What the control looks like: either a screenshot or description
What the variation looks like: either a screenshot or description
Experiment design:
Launch date:
Test location:
Percentage of users tested: if applicable
Minimum sample size:
Time to analyze:
Kill criteria
Results:
Action items:
You don’t need a novel here. Just enough context so anyone on the team could pick it up and understand it. It should take 15 mins to create.
Write it before the experiment starts. Update it when the test ends.
3. Wins & losses tracker
This is your source of truth about what’s worked, and what hasn’t.
Every experiment, whether it succeeded or failed, should have a one-line summary and key takeaway logged here.
The goal isn’t to obsess over the win rate. It’s to build institutional knowledge.
Because otherwise, teams forget. New hires repeat old tests. And everyone wastes time reinventing the wheel.
Every quarter, I like to look back on this doc and ask:
What patterns are emerging?
Where are we consistently seeing wins?
Are we learning fast enough?
The compounding effect of this doc is enormous.
When it’s done well, it becomes a competitive advantage.
You don’t need a giant sample size to run great experiments
You just need signal and a way to validate (or invalidate) your ideas quickly.
You might be tempted to run some paid traffic or even do some outbound prospecting to increase your sample size. But those traffic sources convert at extremely low rates (compared to inbound and other channels) and this often exacerbates this feedback challenge even more.
When traffic is low, qualitative inputs become your best asset. Support tickets, sales calls, user session recordings, customer interviews, churn survey responses - these are great sources.
Use them to identify patterns and surface areas of opportunity. But just because you're using qualitative inputs doesn't mean the tests should be scrappy. You still need process, prioritization, and rigor. Run them through the same brainstorming and prioritization process as high-volume tests. You still want to pick the highest-leverage opportunities.
When it’s time to test, don’t wait for statistical significance (because in a low-volume environment, you'll be waiting forever). Instead, validate with user feedback.
My teams did this constantly in the early days. We’d identify an area of opportunity, design a few options, and use a user testing platform to gather feedback. We’d ask specific, conversion-focused questions to understand whether users noticed the change, understood the value, or were more likely to take action.
If you're at a low-volume environment, this can be your superpower. You don’t need a massive sample size, just clear signals and good instincts.
If you’ve made it this far in the article, you know more about building a high-impact experimentation program than most teams.
You’ve got the frameworks, the docs, and the mindset to start running faster, smarter, more impactful tests.
Whether you’re leading a small team or scaling a $100M org, the same principles apply: Find your bottlenecks. Brainstorm impactful ideas. Prioritize with discipline. And build the habits that turn experiments into momentum.
If you found this helpful, I put together a free 5-day email series that walks you through how to avoid the most common mistakes when building and running growth experiments.
It’s packed with the lessons I wish someone had handed me when I first started out.
If you want to save yourself (and your team) a lot of wasted time, you can sign up here.
Very helpful article for unlocking early-stage analysis-paralysis.
100%. Growth isn’t magic. It’s systems thinking with a short feedback loops.